AI for CxOs: The Hidden Knowledge Ecosystem Inside Your Organization
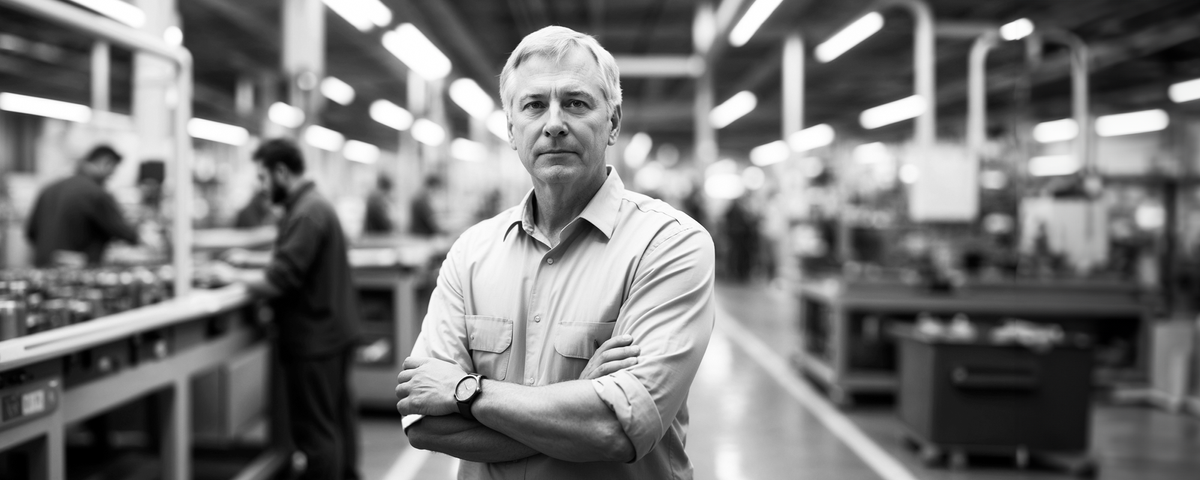
When an experienced employee leaves, they don’t just leave an empty chair—they take years of nuanced knowledge and problem-solving instinct that can’t be easily replaced. Organizations often try to address this through standard training, hoping new hires will “catch up” over time, but research shows that it takes an average of six to twelve months for a new hire to reach peak productivity in a role, and that’s only for documented processes. Tacit and implicit knowledge—those instinctual adjustments honed over years—are nearly impossible to replace through training alone.
This expertise loss goes beyond just procedures; it affects efficiency, quality, and even innovation. In fact, according to a study by the International Journal of Knowledge Management, companies that fail to capture critical knowledge risk productivity dips of up to 25%. Expertise isn’t just surface-level knowledge; it’s the combined effect of tacit and implicit insights that make operations resilient. Companies that take expertise capture seriously—and ground it in contextualized AI—stand to preserve not only knowledge but a competitive edge.
AI can offer tremendous value, but without context, it’s essentially like a new hire with no on-the-job training—clueless about the nuances that keep things running smoothly. A report by Deloitte reveals that 60% of companies struggle to implement AI effectively due to a lack of alignment with business context. For AI to capture real expertise, it has to operate within the specific workflows, terminology, and unique challenges of the organization. This means grounding AI in the environment where expertise naturally thrives.
Imagine an AI system observing how experienced technicians in a manufacturing plant “listen” to machinery, picking up on subtle cues that signify maintenance needs. Or consider a customer service AI that learns when and how to break from a script to address a customer’s concerns genuinely. Contextualized AI isn’t just learning routines; it’s amplifying the unique knowledge that defines your organization’s effectiveness.
A contextualized AI system transforms expertise into a resource that adapts to evolving conditions, making the organization more resilient and adaptive. When AI is grounded in the day-to-day realities of the workplace, it doesn’t just learn facts—it learns how to navigate complexity.
Unpacking Tacit and Implicit Knowledge: The Real Backbone of Expertise
Explicit knowledge—the kind you can document—is straightforward, but tacit and implicit knowledge are far more valuable yet elusive. Tacit knowledge is the “know-how” that employees develop over years, like a gut feeling about the right way to handle a tricky situation. Implicit knowledge is even more subtle: it’s the minor, instinctual adjustments employees make without conscious thought.
A study published in Harvard Business Review notes that over 80% of knowledge within an organization is tacit, which means it exists mainly in the heads of experienced employees and rarely gets documented. That’s where contextualized AI becomes invaluable. By observing and learning from real-world interactions, AI can capture these deeply ingrained insights and make them available to the entire organization.
Consider a hospital setting where nurses make critical care decisions based on both protocol and years of experience with similar cases. A contextualized AI system could observe these interactions and “learn” when deviations from protocol lead to better patient outcomes, preserving that insight as part of a living repository of best practices. This kind of AI-driven expertise capture moves beyond mere data collection; it builds an adaptable knowledge base that helps teams function at their best, even as seasoned employees leave.
How to Capture Expertise with Contextualized AI
Capturing expertise goes beyond recording processes—it’s about preserving the intangible insights that make your organization effective and resilient. Here’s how to build a contextualized AI system that doesn’t just collect data but actively learns from the expertise that drives your business.
- Pinpoint Critical Knowledge Areas: Identify where expertise is most vital. These could be roles or processes where experience is essential to quality or customer satisfaction. For example, in manufacturing, expertise may revolve around machine maintenance or quality control, while in customer service, it’s about handling nuanced client interactions. Prioritize areas where expertise loss would be hardest to replace.
- Conduct Knowledge Mapping Sessions: Gather insights from experienced employees through targeted sessions designed to reveal their thought processes and instinctual adjustments. Instead of a generic Q&A, focus on scenarios where they’ve had to “think on their feet.” Ask questions like, “What signals do you look for before making a decision?” or “Are there exceptions to the rules that help you get better results?” These insights can reveal the unspoken “whys” that guide critical decisions.
- Train AI with Contextual, Scenario-Based Data: Feed AI with data that goes beyond standard procedures and includes real-world scenarios where employees adapt or problem-solve in real-time. Let the AI observe employees handling complex situations—such as troubleshooting a malfunctioning system or managing a high-stakes client call. This way, the AI learns not only what’s done but how and why specific actions are taken.
- Implement Feedback Loops for Continuous Learning: Just like employees learn and adapt over time, so should your AI. Create a feedback loop where employees can provide input on AI-generated insights or recommendations, fine-tuning the system’s understanding of complex or nuanced situations. For example, if a recommendation doesn’t align with on-the-ground realities, employees can flag it, helping the AI refine its decision-making patterns to reflect actual needs.
- Build Accessible Knowledge Resources: Organize captured insights into usable formats, such as digital playbooks or interactive training modules. These resources should be easily accessible, allowing employees—especially new hires—to tap into the expertise of seasoned staff instantly. For instance, a technician troubleshooting a system can reference a playbook embedded with years of real-world problem-solving insights, while a customer service rep can review tips from top-performing agents.
With these steps, your organization creates an AI-powered system that captures expertise not as static data but as a living, learning resource. Contextualized AI isn’t just a “smart” tool; it’s an adaptive partner, reflecting and preserving the instincts, judgments, and experiences that define your company’s success.
The Role of Audio Recordings in Expertise Capture
Audio recordings bring a new dimension to capturing expertise, especially when it comes to the hard-to-document insights that drive effective decision-making. By preserving conversations, explanations, and real-time problem-solving, audio recordings help contextualized AI systems capture and learn from the nuances that written documentation often misses.
- Capturing Real-Time Problem Solving: Recording employees as they work through complex tasks captures not only their actions but the thought processes and reasoning that guide those actions. For instance, a technician explaining each step in diagnosing a machine issue reveals more than just steps—it captures the underlying logic, the “why” behind each choice. These insights, rich in context, are crucial for an AI system to truly understand expertise beyond a checklist.
- Preserving Tone, Emphasis, and Nuance: Audio captures details that written documents overlook, like tone, emphasis, and pacing. A calm, methodical explanation might signal routine troubleshooting, while urgency or caution could indicate a more complex issue. These auditory cues provide additional layers of context that help AI systems understand the different levels of response required in various situations. This nuance helps AI not just “learn” from experiences, but also “sense” when certain decisions should be applied.
- Creating a Virtual Mentorship Library: Imagine a repository of audio recordings from top-performing employees explaining how they handle tough calls, adapt to client needs, or troubleshoot complex issues. When paired with AI, these recordings become a virtual mentorship library, accessible to employees across the organization. New hires can hear experienced colleagues’ solutions in action, giving them practical insights and confidence to handle challenges. It’s like having access to decades of expertise on demand.
Integrating audio into your expertise capture strategy allows contextualized AI to absorb the subtleties of real-world problem-solving, preserving the authentic voices and reasoning of your team. This approach doesn’t just document knowledge—it brings it to life, creating a multi-dimensional resource that captures both the content and context of valuable expertise.
Building a Sustainable Expertise Ecosystem
Capturing expertise is one thing; sustaining it as a living, adaptable resource is another. Contextualized AI offers the chance to create a sustainable expertise ecosystem—a knowledge network that grows and evolves with your organization. Instead of static archives, you’re building an ecosystem that continuously learns, adapts, and keeps knowledge relevant.
A sustainable expertise ecosystem works as a dynamic, knowledge-sharing loop. As employees interact with contextualized AI, the system not only preserves their insights but also learns from each interaction, refining and expanding its knowledge base. This allows expertise to evolve naturally as new challenges arise, technologies advance, and employee practices adapt. Over time, the ecosystem becomes a living repository of real-time insights, accessible and applicable to every level of the organization.
In this model, expertise isn’t just preserved when employees leave or retire; it’s continually renewed, providing a resource that scales with the organization. A sustainable expertise ecosystem also enables cross-functional learning, where insights from one department can be shared and applied across others. For instance, knowledge captured from experienced technicians in manufacturing can be leveraged by product development teams to improve design or efficiency, fostering a culture of shared knowledge and innovation.
Contextualized AI isn’t just a “tool” within this ecosystem; it’s an adaptive partner that captures not only the “how” but the “why” behind actions. This creates a resilient foundation for decision-making, so that whether you’re onboarding new hires or evolving processes, you have a solid base of adaptable, real-world expertise to rely on. In the end, this sustainable expertise ecosystem empowers companies to keep knowledge fluid, relevant, and accessible, ensuring that the organization doesn’t just retain knowledge but grows from it.
The concept of knowledge creation within organizations isn’t new. Ikujiro Nonaka, a pioneering thinker in this space, developed the SECI model (Socialization, Externalization, Combination, and Internalization), which explains how knowledge flows and transforms within companies. Nonaka emphasized the importance of tacit knowledge—those deeply ingrained insights and instincts that employees develop through experience. He argued that tacit knowledge is invaluable and often irreplaceable, and its capture and sharing are crucial to creating a “knowledge-creating company.”
Contextualized AI brings Nonaka’s model into the digital era, providing a means for organizations to capture and distribute tacit knowledge in real time. In Nonaka’s model, tacit knowledge is made explicit (Externalization) and combined with other knowledge sources to create a richer, more comprehensive resource. With contextualized AI, organizations can now capture this process on a continuous basis, creating an environment where insights and expertise are not only preserved but expanded and adapted.
Consider Nonaka’s Socialization phase, where knowledge is shared through shared experiences and interactions. Traditionally, this happens in person or through mentorship. Contextualized AI replicates this by learning from employees’ real-world interactions and decisions, capturing unspoken know-how and distributing it across the organization. The AI essentially acts as an active participant in Nonaka’s model, constantly observing, learning, and updating its “understanding” based on new inputs and evolving conditions.
With contextualized AI, companies can finally operationalize Nonaka’s vision of a knowledge-creating organization. The AI becomes a dynamic repository of knowledge, ensuring that the organization’s culture of expertise isn’t lost or diluted over time. Instead, expertise evolves in line with Nonaka’s principles, transforming tacit knowledge into a shared, adaptable resource. This approach allows companies to build a resilient foundation of knowledge that can adapt to new challenges, maintain continuity, and foster a culture where learning is continuous and knowledge is always within reach.
Why Ignoring Expertise Capture Isn’t an Option
Investing in contextualized AI isn’t just about continuity; it’s about ensuring your organization remains adaptable and resilient. AI systems that capture, store, and evolve with the nuanced knowledge within your organization create a legacy of expertise that future employees can tap into immediately. Companies that prioritize this now position themselves not only to retain their competitive edge but to thrive in times of change.
According to a recent survey by Deloitte, companies that invest in AI-driven knowledge management see up to a 20% reduction in time spent on routine tasks and report improved decision-making quality across departments. In other words, contextualized AI isn’t just a safety net; it’s a tool for growth and efficiency, providing a clear ROI while building a culture of continuous learning.
So, the question is no longer “Can we afford to invest in expertise capture?” but rather, “Can we afford not to?” In a rapidly changing world, companies that embed contextualized AI into their knowledge ecosystems won’t just survive—they’ll set the standard. Now is the time to preserve your most valuable asset: the collective expertise that defines who you are and how you operate. Ignoring expertise capture isn’t standing still; it’s a step backward.
This exploration in expertise is by Eric A, a simulated digital persona designed to explore the future of knowledge in the enterprise. Content AC-HA.
Comments ()